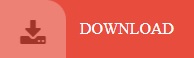
To make it easier to refer to the module in our script, we abbreviate it as plt. We specify the module we wish to import by appending. Scatter.py import matplotlib.pyplot as plt If not, you will receive an error message:
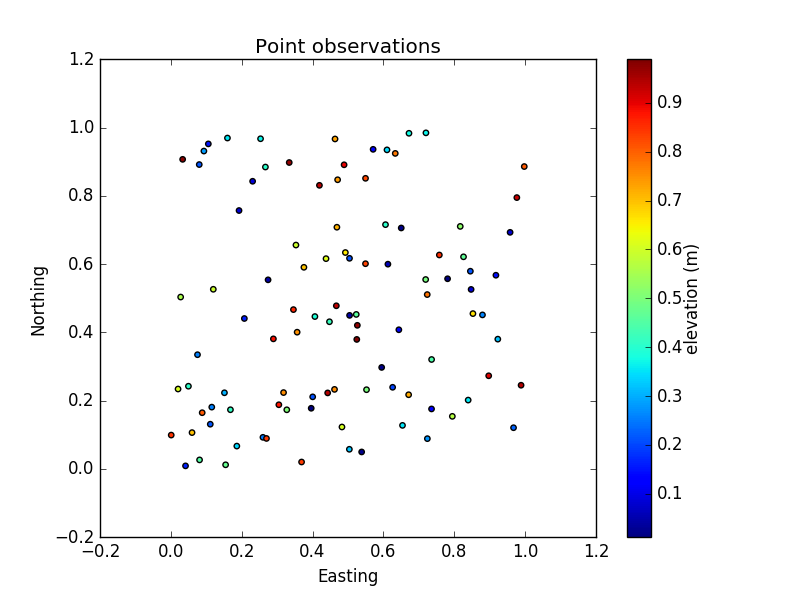
If matplotlib is installed, this command will complete with no error and we are ready to go. In the command line, check for matplotlib by running the following command:

Step 1 - Importing matplotlibīefore we can begin working in Python, let’s double check that the matplotlib module is installed. If this is not the case, you can get set up by following the appropriate installation and set up guide for your operating system. When you are finished with this tutorial, you’ll be able to plot data in Python! Prerequisitesįor this tutorial, you should have Python 3 installed, as well as a local programming environment set up on your computer. We’ll go through generating a scatter plot using a small set of data, adding information such as titles and legends to plots, and customizing plots by changing how plot points look.
#Add point to scatter plot matplotlib how to
Given the importance of visualization, this tutorial will describe how to plot data in Python using matplotlib. It is good to keep in mind that visualization is a blend of art and science. Each plot presents data in a different way and it is often useful to try out different types of plots before settling on the most informative plot for your data. It allows you to generate high quality line plots, scatter plots, histograms, bar charts, and much more. Hunter and since then has become a very active open-source development community project. We’ll be using the 2D plotting library, matplotlib, which was originally written by John D. Whenever we are working with data, visualization is often a necessary part of the analysis. Visualization is a quick and easy way to convey concepts in a universal manner, especially to those who aren’t familiar with your data.

Visualizing the data, by plotting the selling price versus age, could definitely shed some light on the relationship that exists between the two. Then it would become pretty difficult to understand how age affects price. However, say you wanted to use data from the entire town of 500 houses. If your data included 1 block of 5 houses, it wouldn’t be too difficult to get a sense of what is going on. Data visualization is a useful way to help you identify patterns in your data.įor example, say you are a real estate agent and you are trying to understand the relationship between the age of a house and its selling price. Often a data set will include multiple variables and many instances, making it hard to get a sense of what is going on.
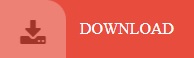